What is the problem?
In 2010, the Mexican government introduced a performance evaluation system across all departments to keep track of the impact of public programming and spending. The system, based on the Logical Framework methodology from USAID, is intended to increase accountability and improve results of public service delivery. Over the years it has been used by civil servants across the government to keep track of their progress against a set of indicators unique to each public spending initiative. The evaluation includes open text entries where civil servants write a brief justification to account for goals that weren’t met. To date, there has been no analysis of this rich text dataset which spans many different public services over the last ten years – despite its potential to surface novel insights about common barriers to implementation which could improve the design of future programs.
What does the Accelerator Lab plan to do?
The Lab is mining the text entries using NLP to cluster and rank dominant themes across this vast public service dataset. By training an AI model to compare text entries with a set of predefined common causes and identify novel themes, they’re aiming to improve the reporting process for civil servants in the future. The algorithm is enabling them to analyze and make use of civil-servant generated data that’s otherwise too unstructured for centralized analysis. Eventually the team hopes to build a hybrid collective intelligence model, where the quality of evaluations is improved by combining NLP classification and inputs from civil servants in real time.
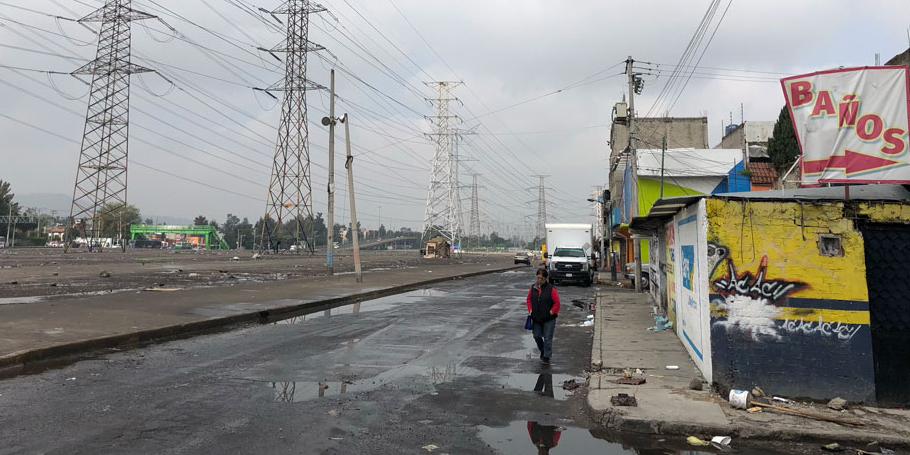
What does this experience tell us about collective intelligence design?
Tackling a complex problem, like improving the effectiveness of government policy implementation, isn’t something for which there is a single silver bullet solution. The text-mining process will help the government to extract lessons at a speed and scale that was not previously possible. However, it will need to be implemented alongside other efforts (such as changes to the user interface of reporting software) to make the existing program evaluation system more effective. This will help to ensure that the insights from the model are integrated into the machinery of government and affect future program design. The Lab is already considering how to achieve this. Ideas include involving civil servants in verifying the model and developing skills across government teams to enable them to work with the new system in the long term.