Use case 2 Anticipating, monitoring and adapting to systemic risks
A wide range of collective intelligence methods are helping governments and international agencies improve their capacity for early warning and monitoring of, and response to, natural disasters, conflict and epidemics. Collective intelligence is also steadily being harnessed by communities themselves for better preparedness and response.
From citizen science, to web scraped data, crowd forecasting, sensor data and crowdmapping – there are five common applications of collective intelligence methods to help organizations anticipate and respond to systemic risk.
- Citizen science to gather new data for early detection or monitoring of public health threats
- Combining web scraped and/or existing datasets for real-time surveillance of public health threats
- Combining web scraped and/or citizen-generated data to inform crisis response
- Crowd forecasting of epidemics, conflict and geopolitical events
- Crowdmapping (sometimes combined with satellite/sensor data) for community preparedness and response
- Strengthen the capacity of all countries, in particular developing countries, for early warning, risk reduction and management of national and global health risks (3.d)
- Strengthen resilience and adaptive capacity to climate-related hazards and natural disasters in all countries (13.1)
- Reduce violence and related deaths (16.1)
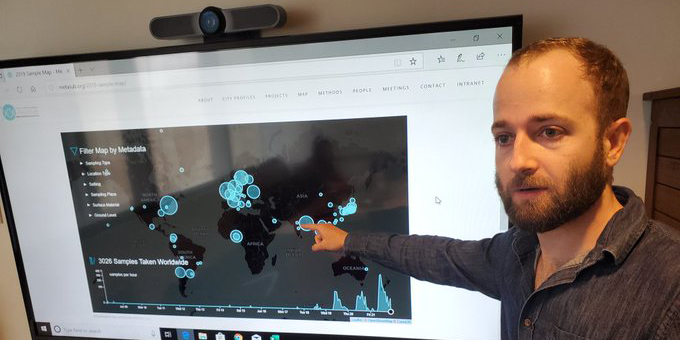
Citizen science to gather new data for early detection or monitoring of public health threats
One benefit of working with distributed networks of citizens to gather data through methods like citizen science, is that they can help to create much needed data about new and emerging issues. Citizen science can also be good for encouraging mass participation and building awareness of an issue.
MetaSUB is a global project to build microbial portraits of urban transit systems. A network of volunteers and scientists takes swabs from trains and escalators. They identify the pathogens they find and any markers of antibiotic resistance. The microbial surveillance carried out by the MetaSUB community in over 100 cities helped add to research which found that the longer COVID-19 was on a surface the less likely it was to make someone sick. Increasingly AI is being used to find patterns amongst the mountains of data generated by this project. In the future, it may even help make predictions, or spot future pandemics much earlier.
Another project that harnesses citizen scientists for disease surveillance is Mosquito Alert – an app where volunteers notify scientists of the presence of mosquitoes, or their breeding sites, by uploading photos and location information. Data is validated by scientists and then published on a publicly-available map. As well as helping scientists, the data is used by public health managers from a variety of Spanish cities to monitor and control mosquito populations. As of December 2020, the app had over 96,000 registered citizen scientists taking part in the project. And over 13 per cent of the one billion observations contained in the Global Biodiversity Information Facility are from citizen science data generated through Mosquito Alert’s app.
Combining web scraped and/or existing datasets for real-time surveillance of public health threats
Collective intelligence methods like web scraping and combining different data sources are making it easier to monitor public health threats in real-time. The COVID-19 crisis has seen these methods applied to the creation of a number of dashboards, interactive maps and visuals that track the virus’ spread and its impact. The tracker developed by John Hopkins University has become the primary source of information for journalists and people around the world to monitor the spread of the virus.
An example predating the COVID-19 pandemic is HealthMap, established in 2008, which utilizes online information for disease outbreak monitoring and real-time surveillance of emerging public health threats. An automated process brings together disparate data including online news, eyewitness reports and official reports to monitor, visualize and disseminate online information about emerging diseases in nine languages. Its data is used by the World Health Organization, the US’ Centers for Disease Control and Prevention, and the European Centre for Disease Prevention and Control, among others. But unlike other systems that target only public health officials, it also has an app that is geared towards citizens, allowing them to see outbreaks in their neighborhood.
Increasingly, however, collective intelligence initiatives are moving beyond monitoring epidemics to trying to predict them using machine learning models. AIME (Artificial Intelligence in Medical Epidemiology) is an AI platform to predict mosquito-borne disease outbreaks. During tests of its dengue prediction system, it was successful in predicting the next outbreak of dengue fever up to three months in advance. To do this, the platform combines real-time data generated by doctors, who send in notifications of dengue cases, with existing datasets of variables that influence the spread of dengue — from local terrain and elevation to roofing types and thunderstorms. From these, the system then predicts where the next outbreaks will be within a 400-metre radius, and with 81 per cent accuracy. The platform is now used in Malaysia, Brazil and the Philippines to help healthcare providers manage and curb outbreaks.
Combining web scraped and/or citizen-generated data to inform crisis response
The same web scraping methods used for disease surveillance are also being used to provide humanitarian agencies and emergency responders with faster and more relevant information in the immediate aftermath of a crisis. Combining existing datasets with locally-generated contextual information from citizens helps those planning emergency responses.
MIND (Managing Information for Natural Disasters) is a tool developed by UN Global Pulse to better inform humanitarian response planning and logistics after natural disasters. It’s built on an automated data pipeline that analyzes several non-traditional datasets all in one place. The tool combines information from Twitter and Google searches in the affected areas, as well as information from Wikipedia and OpenStreetMap about the location, casualty reports from news sources, and data from the International Aid Transparency Initiative, showing details about projects and organizations in the affected areas. MIND is still a research prototype that is openly available for user testing.
eBayanihan – a mobile and web-based participatory disaster management system which was developed and piloted in the Philippines during 2014-15 – represents a different approach. Unlike MIND, it did not scrape data from social media feeds of affected citizens, but allowed them to post reports of damage directly. They could also provide an initial needs assessment following incidents and request skills, resources or volunteers for the response. Machine learning algorithms helped model and validate these crowdsourced reports –categorizing and visualizing them. It provided real-time notifications to people managing response clusters. Unfortunately, it’s unclear to what extent the system is still in use or how it’s integrated into government disaster management.
Crowd forecasting of epidemics, conflict and geopolitical events
Triggers for some crises are difficult to predict in advance due to their rare occurrence. With new pandemics or conflict, the lack of historical data can make statistical predictions or machine learning models unreliable. To address gaps in these methods, some organizations are employing another collective intelligence method called ‘crowd prediction polling’ or crowd forecasting. These ‘wisdom of the crowd’ forecasts are calculated by aggregating many individual judgments about the likelihood of events. Past research has even suggested that a non-specialist crowd can predict geopolitical events more accurately than individual analysts.
For example, the Early Warning Project produces a ranked list of more than 160 countries, based on their likelihood of experiencing a mass killing, in order to better target preventative action by governments and charities. It does this using a combination of crowd forecasting, expert ranking and a machine learning model. During the first phase, experts in the field take part in an annual comparison survey where they rank pairs of countries according to which is more likely to experience a mass killing. A machine learning model also generates estimates based on more than 30 variables from historic datasets. Seventeen ‘higher-risk’ countries are then tracked using crowd forecasting, producing quarterly forecasts over the course of a year. As forecasters can regularly update their estimates with new information, they’re able to pick up and reflect ‘weak signals’ or sudden changes in the context missed by the other methods.
The Flu Forecasting Center at Carnegie Mellon University also taps into a group of volunteers (both experts and non-experts) for their annual flu tracking system. Each week volunteers log into an online system to review a chart showing the trajectory of past and current flu seasons. They’re then asked to complete the current season’s curve, projecting how many more flu cases there will be over time. The crowd’s aggregate forecast is often just as accurate as the machine learning prediction, trained on over 20 years of data. Over the last few years, the center has fine-tuned this combination of human and machine predictions to forecast the trajectory of the flu with a high degree of accuracy, beating the attempts of many other researchers.
Crowdmapping (sometimes combined with satellite/sensor data) for community preparedness and response
The best collective intelligence initiatives open up data for use by members of the public as well as decision makers – increasing people’s ability to make decisions and power to act. Crowdmapping and GIS are the most commonly applied collective intelligence methods for gathering and sharing information in this way. Some initiatives combine this citizen-generated data with sensor or satellite data for a fuller picture of a situation and how it’s changing.
One of the best known examples is Peta Bencana, which is applying collective intelligence methods to disaster response in the Indonesian capital of Jakarta. It creates real-time flood maps by combining reports from citizens on Twitter with official data on flooding and sensors from around the city. It’s used by the local government to prioritize its flood response and by residents to make informed decisions about how to navigate around the city.
In Tanzania, Ramani Huria helps communities in informal settlements to map residential areas, roads, streams, floodplains and other relevant features – aiming to bring disaster prevention and response to areas that were previously off the map. In 2015, it helped public authorities respond to an unexpected outbreak of cholera, providing detailed information on water points and sanitation data. During the COVID-19 pandemic, the Taiwanese government made data on the nationwide stock of masks publicly available using an open API. This allowed civic-minded developers to create interactive maps showing the real-time distribution and supply of masks. These were used by citizens queuing for masks and enabled the government to address distributional problems.
There are many other successful deployments of crowdmapping globally, even stretching to the Arctic. The SIKU platform combines satellite imagery with reports from indigenous communities in their own languages of weather conditions, ice hazards and wildlife. It helps document the impact of climate change, and is used to share dangerous and changing ice conditions within Inuit communities.