How is AI augmenting collective intelligence for the SDGs?
Most uses of AI in development today are based on machine learning algorithms, which improve their performance based on extracting patterns from very large training datasets. This approach is a great fit for collective intelligence projects, many of which gather or interpret large amounts of human-generated content like images and videos, crowdsourced through smartphone apps and online platforms.
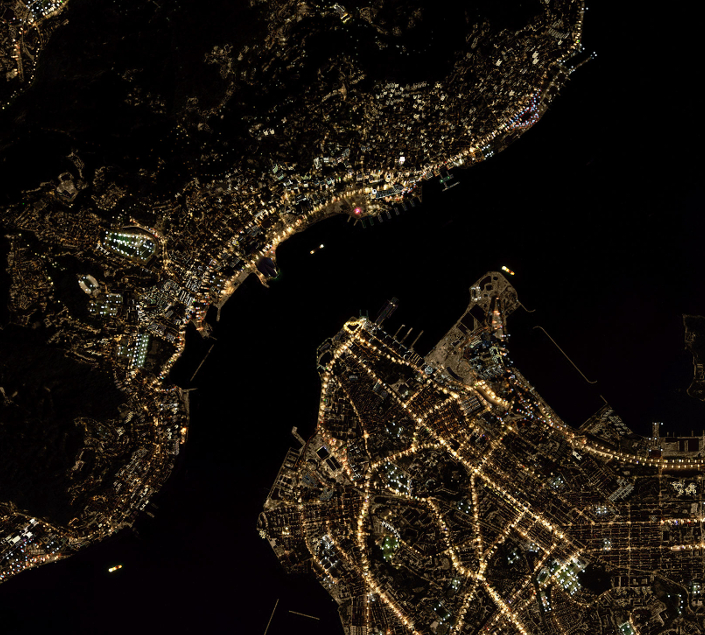
Increasingly AI techniques like natural language processing, machine learning and predictive analytics are being used alongside the most common methods in collective intelligence, from citizen science and crowdsourcing to digital democracy platforms.
At its best, AI can be used to augment and scale the intelligence of groups. In this section we describe the potential offered by these new combinations of human and machine intelligence. First we look at the applications that are most common, where AI is being used to enhance efficiency and categorize unstructured data, before turning to the emerging role of AI - where it helps us to better understand complex systems.
These are the three main ways AI and collective intelligence are currently being used together for the SDGs:
1. Efficiency and scale of data processing
AI is being effectively incorporated into collective intelligence projects where timing is paramount and a key insight is buried deep within large volumes of unstructured data. This combination of AI and collective intelligence is most useful when decision makers require an early warning to help them manage risks and distribute public resources more effectively. For example, Dataminr’s First Alert system uses pre-trained machine learning models to sift through text and images scraped from the internet, as well as other data streams, such as audio broadcasts, to isolate early signals that anticipate emergency events.
2. Organizing human knowledge
To make the most of collective intelligence, it’s important to draw on a diversity of views. But it’s difficult to synthesize hundreds of inputs to extract common themes and priorities, especially when working with non-traditional citizen-generated data. This is often the limiting factor in large scale deliberative or consultation exercises, and here, AI can help. AI methods like natural language processing (NLP) can be used to identify prominent topics and help to cluster the data by meaning. Popular citizen participation platforms like CitizenLab and Decidim are already experimenting with these AI methods and NLP has also been used to identify SDG priorities in the context of a social listening project by Pulse Lab Kampala.
3. Optimizing and prioritizing workflows
Finally, we see examples of AI being deployed alongside volunteers to help them complete microtasks with more consistency and accuracy. For this combination of AI and collective intelligence, the performance gains may need to be carefully balanced with how comfortable volunteers feel about working with AI tools. For example, in 2018 the Humanitarian OpenStreetMap Team (HOT) launched the RapiD tool that uses computer vision to detect roads and terrain features, to help their volunteers complete crowdmapping tasks more quickly during crisis response. The RapiD tool was first used to support mapping in Kerala, India during a flooding event but has since been fully integrated into the HOT platform. Importantly, HOT offered volunteers the opportunity to opt out if they preferred working without AI.
How might AI and collective intelligence help us get even better at working with complex systems?
One significant future opportunity lies in using AI to augment collective intelligence to model scale and complexity. For complex challenges, it can be hard to connect local issues to global problems, or make the case for short-term sacrifices that bring long-term benefits. Methods based on distributed AI are emerging to help us overcome these limits of perception. For example, agent-based modeling and multi-agent systems are being used by decision makers to explore different scenarios and understand the impacts of interventions they plan to make, while generative AI models are helping to visualize long-term impacts of systemic problems like climate change. These emerging AI methods can be used to explore the complexity at the heart of the SDGs.
CityMatrix is a good example of what this looks like in practice. It helps decision makers test out the impact of urban planning decisions. Using many different sources of urban data, CityMatrix creates an interactive simulation of a city environment, powered by agent-based modeling. The tool’s visual interface allows participants to set policy priorities and choose between different options recommended by an optimization algorithm. Variants of the tool have been piloted with a number of cities, including Paris, Boston and Riyadh, but it will take time for these types of models to become a mainstay of collective decision making. Ultimately, tools like CityMatrix may be used to bring together different stakeholder groups across cultural and social divides to collaboratively explore complex issues while engaging in deliberation about competing values, trade-offs and priorities. There are promising signs of progress from the development sector. In 2020, the UN announced that it was planning to test out a simulation tool for policy prioritization with governments in Latin America.