What is the problem?
Serbia has one of the 10 fastest-shrinking populations in the world, with an 18.9 per cent decrease in population predicted between now and 2050 and approximately 120,000 people reportedly leaving the country each year to pursue work opportunities in other countries. This pattern of depopulation has a significant impact on the country’s labor market, through the loss of the youngest people from the workforce and a growing deficit among both high- and low-skilled workers. By better understanding the education and professional opportunities sought by the approximately four million Serbians living abroad, the government aims to develop policies to stimulate their return in order to bring their networks and skills back home. Governments struggle to track the dynamics of modern economic migration in a precise way, especially when national statistics offices rely on traditional and infrequent data collection practices such as the census.
What did the Accelerator Lab and partners do?
To overcome the limits of traditional labor market data, the Accelerator Lab used collective intelligence to map and understand the drivers of outward migration in real time. The team integrated novel data (made available by the World Bank) about Serbian users of LinkedIn and Serbian language work-related Google searches. The LinkedIn data was anonymized to preserve privacy, but contained valuable information about the locations, industries and skills of Serbian professionals.
The Lab also launched a data challenge with a US$50,000 prize to surface other unusual data sources that could reveal further insights about the labor market and depopulation, or triangulate the findings from the other data. Winning ideas included using Facebook Ads to identify emigration trends, and analyzing mobile phone data to better understand activity, connectivity and mobility of people within Serbia.
Figure 4
Industries with Highest Out Migration from Serbia 2018 - Net Change
Industries Gaining / Losing - The net gain or loss of members from another country working in a given industry divided by the number of LinkedIn members working in that industry in the target (or selected) country, multiplied by 10,000.
What was the benefit of using collective intelligence for this issue?
The LinkedIn data has helped the team to identify trends in the skills lost and industries affected by relocation of working-age Serbians. The data revealed that research, international affairs and financial services sectors were heavily affected, while some of the top skills lost included genetic engineering and AI. Through Google search data, the team were also able to uncover microtrends in the geographical distribution of the Serbian diaspora. For example, the data highlighted a significant outflow of doctors and healthcare practitioners from Serbia to Germany and Austria. Emerging findings from the data challenge have confirmed that Germany and Austria were the main destination countries for professionals, while Montenegro, Slovenia and Croatia accounted for migration of seasonal workers.
Figure 5
Main destination countries for professionals and seasonal worker from Serbia
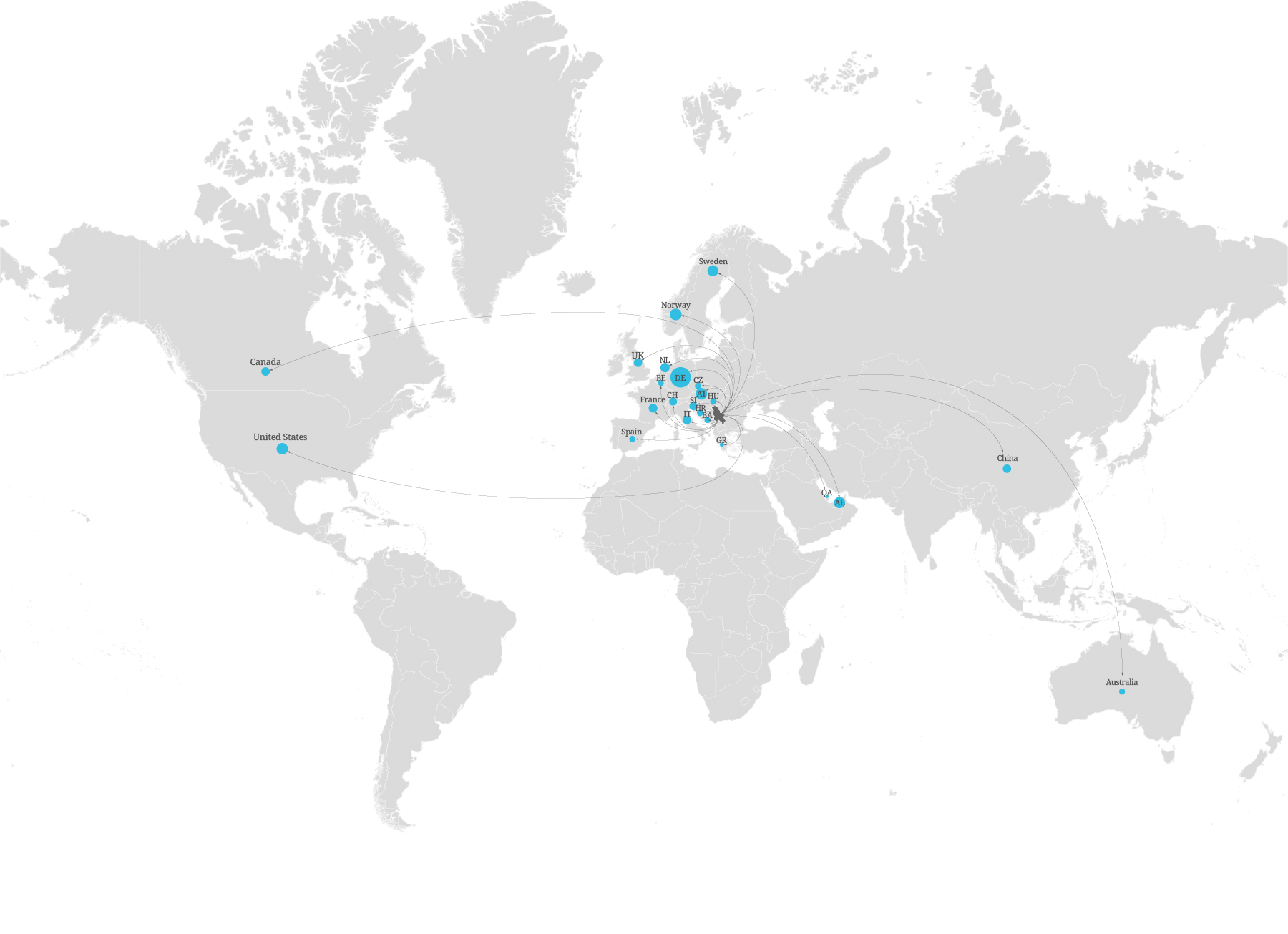
What next?
The team are currently exploring how these insights can form part of a broader portfolio to shape concrete policy interventions. An example of this is using multilateral agreements and incentives to encourage the reciprocal exchange of medical professionals between Serbia to Germany and Austria. They’re also planning to create a centralized platform to share the results from the data challenge. The site will feature multiple near real-time dashboards on skills and sector trends to inform the decisions of job seekers and the policy makers working on skills retention.
What does this experience tell us about collective intelligence design?
When tapping into private sector datasets, it’s important to remain flexible and anticipate what might be possible with a ‘minimum viable product’ approach. The Lab spent many months exploring publicly available LinkedIn datasets, which were limited in their granularity. Higher-level aggregation of data helps to protect the privacy of users of the platform, but also prevents the disaggregation of results by certain important characteristics, such as gender and age. By refining their research questions, they were able to get the most value from the available data whilst avoiding lengthy and expensive negotiations with a private company. This highlights the challenge of undertaking collective intelligence projects that draw on both proprietary and publicly available data.