What is the problem?
The informal sector accounts for close to 90 per cent of the economy in Zimbabwe. Due to its invisibility, informal economic activity isn’t well understood, which makes it difficult to mitigate against market disruptions. For example, the country’s food production and distribution industry has a high proportion of informal actors including growers, distributors and sellers. In the wake of the COVID-19 pandemic, Zimbabwe faced sudden food shortages. But it was difficult to identify the exact sources and drivers of this disruption due to data gaps about the ecosystem that supports food production.
What did the Accelerator Lab and partners do?
To draw attention to the informal economy’s contribution to food supply chains, the Lab used a combination of non-traditional data sources and qualitative insights from informal actors – to identify trends, market vulnerabilities and associated behaviors. The Lab worked with three major national trade associations (Knowledge Transfer Africa, Bulawayo Vendors and Traders Association, and the Zimbabwe Chamber of Informal Economy Associations) to obtain historical datasets on the volume and pricing of food supplies to informal markets. Although the associations held many datasets about the daily changes to market prices between them, the data was not interoperable and hadn’t previously been combined. In partnership with Magic Leap and Flow Immersive, the Lab combined this data into an immersive virtual reality data visualization to help communicate results more effectively to decision makers.
The team also enriched this data with insights from a qualitative survey of more than 3,000 vendors, distributors and suppliers from across the country. Using the interactive voice tool, Viamo, they sent voice response questionnaires to vendors in three different cities in Zimbabwe (Mutare, Harare and Bulawayo), as well as several smaller border towns.
What was the benefit of using collective intelligence for this issue?
By combining these novel datasets, the team were able to analyze trends and fluctuations within the entire food supply ecosystem in Zimbabwe. For example, they identified a consistent annual trend of a drop in sales in January, which emerged from surveying the daily market transaction data at the national level. In 2020, unlike previous years, this seasonal decrease continued into March and April due to the introduction of lockdown measures, which further exacerbated the loss of earnings experienced by vendors. The data also helped the team to quantify the market impact of droughts caused by climate change on important crops, such as maize.
By making the data from different associations interoperable, the team helped to establish a new standardized data infrastructure for understanding the value chain of fresh produce. This data capture system is the only overview of the food production system in all its complexity. It provides a foundation for trade associations and politicians to continue tracking activity in the future.
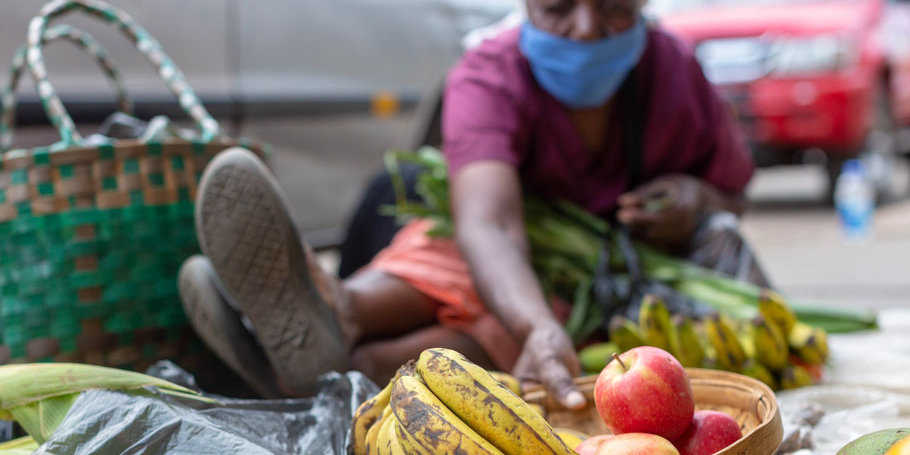
What next?
The team shared the visualized data with the trade associations, vendors and policy makers to help them better anticipate regular changes in the market, as well as emerging trends linked to crises like COVID-19 and climate change. This has led the Lab to design a broader portfolio around urban food poverty, NextGenCities, together with government, local councils and vendor associations. As part of this program, they plan to make the data capture system they developed for tracking daily market prices openly accessible.
They’ve also shared their experience of working on this issue with Lab colleagues in Eswatini and Botswana to explore scaling the model to neighboring countries.
What does this experience tell us about collective intelligence design?
It’s difficult to build communities or establish new networks from scratch, and often it isn’t necessary. Partnering with trade associations helped the Lab to gain the trust of informal workers who they struggled to engage with in the initial phases of the project. The partnerships also extended their reach geographically, allowing them to generate insights using national scale data across a range of different locations. For collective intelligence to succeed, tapping into existing coalitions and networks generates meaningful insights and change.